Table of Contents
TogglePharma 4.0 represents a transformative cultural shift toward human-centric, digitally connected workflows in Pharma Manufacturing. The industry is embracing this shift by empowering itself with information systems and making laboratories and factories smart. These upcoming changes demand that pharma manufacturing systems must be able to implement corrective actions and instantly respond to demand fluctuations.
With digitalization at the forefront of Pharma 4.0, the transition to a paperless facility is inevitable. The idea is to connect various parts of the value chain and create new levels of adaptivity and transparency for a digitalized system. The concept builds on process analytical technology and ‘Data Integrity by Design’. Thus, moving the focus to real-time monitoring using connected systems. Pharma 4.0 enables a truly agile manufacturing system where processes self-adjust based on the collected data.
For quality-driven organizations, evaluating the current procedures on past data is important. The goal is to derive maximum efficiency and value from the available data. The focus is also on systems that aid in achieving data integration and analysis across the organization. However, to utilize the latest data analysis techniques such as Artificial Intelligence (AI) and Machine Learning (ML), data must be at the heart of this entire system.
The Untapped Potential of Data
AI and ML are major tech elements that are transforming the industry. Contributing largely to commercialization, early drug discovery, and clinical trial design, these tech elements are evolving in new ways every day. This evolution leads to inevitable challenges. Many pharmaceutical companies are still discovering and testing new potential for these analytical tools. In this process, they come across some challenges.
- Data Quality
Pharmaceutical companies are highly data-driven and in the presence of biased data, actions and results depicted by AI shall be influenced. Data governance poses a major challenge in this aspect. This may raise problems when it comes to handling targeted results. AI can learn better from more specific and defined data sets. Companies should pick vendors who assure complete data security with a data encryption system. Systems must be built to ensure data integrity and meet the principles of ALCOA Plus as per GxP guidelines.
- Automation
Although AI and ML have made processes a lot easier, there are apprehensions among users about their abilities to automate complex processes. Until they gain complete trust in these tech elements, the need for human intervention prevails. Robust manufacturing and quality solutions can eliminate these insecurities by setting a foundation for digital transformation efforts.
- Data Silos
Organizations have successfully digitalized most of their manual processes. However, quality efficiency is still a faraway goal. Huge data sets collected from various systems like LIMS, EBR, ERP, DMS, excel, etc. in the form of COAs, stability testing reports, CAPA analysis reports, dossiers, etc. are lying separately as data silos. Systems like EPIQ QMS aim to address the rising data silo issues and are clearing the way by offering in-depth integrations.
Looking Beyond Disruptions
Digital solutions offered by disruptive technologies can help manufacturers in many ways. Apart from preventing equipment breakdowns and ensuring process consistencies, they can contribute to overall production optimization. Advanced analytics such as AI and ML can help them prevent manufacturing losses, increase equipment reliability, improve the quality and lifecycle of drugs, and eventually reduce capital expenditure. By identifying process degradation and its impact on final product quality to reducing lab testing lag times, these tech elements offer a competitive advantage to pharmaceutical companies.
Predictive Maintenance and Process Analytics
Predictive maintenance uses advanced analytics to raise alerts by identifying the signs of impending equipment failure. This allows drug manufacturers to avoid unplanned failures. Easy-to-use predictive maintenance software captures and analyzes data quickly while also identifying anomalies at an early stage. Process analytics evaluates complex variables in batch production and determines critical ones to drive greater yields. Thus, these tech elements (AI and ML) which are also termed disruptive technologies, safeguard the security of supply, control costs, and optimize production.
Embrace the ‘Holistic Control Strategy’
Pharma 4.0 promotes an integrated approach to ongoing change and product/process life-cycle management. Holistic control strategy evolves as a digital catalyst for the supply chain – including the manufacturers, marketers, stakeholders, and patients. It drives manufacturing process accuracy and efficiency, improves quality, and ensures sound, real-time decision-making. Driven by the Pharma 4.0 operating model, the strategy enables a holistic value network structure. The holistic approach goes beyond the process variability checks and drug development parameters. It includes all the events that occur during the entire product lifecycle, the supply chain, and patient needs and conditions.
Data Integrity by Design
This Pharma 4.0 imperative aligns with the new-age shift from application integrity to data integrity. Regulators have recognized the need for prospective data quality control to ensure validation procedures are sustained in such a highly versatile environment. Manufacturers must take the next step to make data integrity intrinsic and collaborative, updating their validation procedures to enable rapid product life cycles.
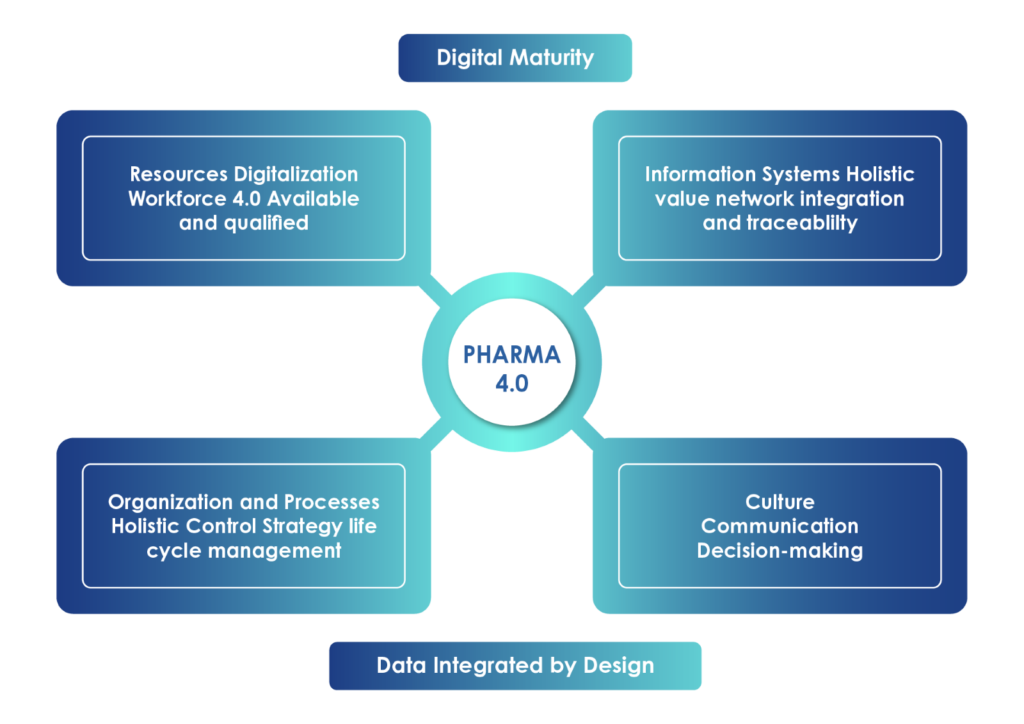
Becoming a ‘Good Data’ Organization
Good data is the crux of the foundation for Pharma 4.0. Robust systems that are interconnected and well-integrated with instruments and machines are an absolute necessity to be ready for future disruptions. Pharma manufacturing is a constantly evolving industry and is growing increasingly complex with time, so knowing how to quickly adopt these processes is key. By understanding how to increase reliability, effectiveness, and output while maintaining quality with advanced analytics based on good data, companies will hold a sustainable competitive advantage while protecting their bottom line and safeguarding patients’ lives.